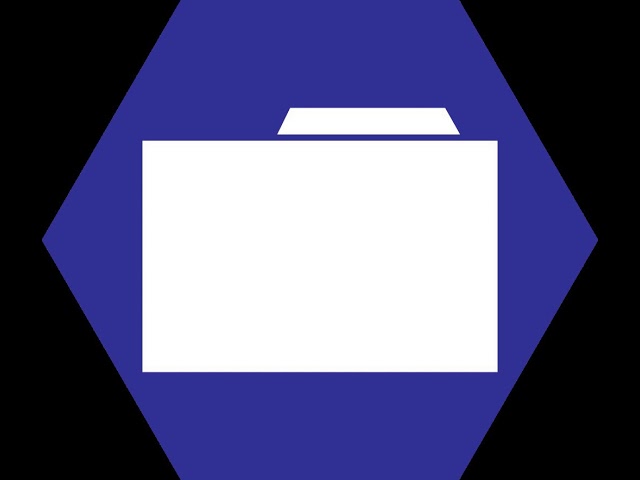
00:00:58 1 Definition
00:02:56 2 Examples
00:03:25 2.1 Sample variance
00:17:08 2.2 Estimating a Poisson probability
00:19:06 2.3 Maximum of a discrete uniform distribution
00:20:04 3 Median-unbiased estimators
00:21:03 4 Bias with respect to other loss functions
00:22:02 5 Effect of transformations
00:23:01 6 Bias, variance and mean squared error
00:24:59 6.1 Example: Estimation of population variance
00:26:27 7 Bayesian view
00:27:26 8 See also
00:31:21 9 Notes
00:32:20 10 References
Listening is a more natural way of learning, when compared to reading. Written language only began at around 3200 BC, but spoken language has existed long ago.
Learning by listening is a great way to:
- increases imagination and understanding
- improves your listening skills
- improves your own spoken accent
- learn while on the move
- reduce eye strain
Now learn the vast amount of general knowledge available on Wikipedia through audio (audio article). You could even learn subconsciously by playing the audio while you are sleeping! If you are planning to listen a lot, you could try using a bone conduction headphone, or a standard speaker instead of an earphone.
Listen on Google Assistant through Extra Audio:
Other Wikipedia audio articles at:
Upload your own Wikipedia articles through:
Speaking Rate: 0.8491775158449761
Voice name: en-GB-Wavenet-A
"I cannot teach anybody anything, I can only make them think."
- Socrates
SUMMARY
=======
In statistics, the bias (or bias function) of an estimator is the difference between this estimator's expected value and the true value of the parameter being estimated. An estimator or decision rule with zero bias is called unbiased. In statistics, "bias" is an objective property of an estimator. Unlike the ordinary English use of the term "bias", it is not pejorative even though it's not a desired property.
Bias can also be measured with respect to the median, rather than the mean (expected value), in which case one distinguishes median-unbiased from the usual mean-unbiasedness property. Bias is related to consistency in that consistent estimators are convergent and asymptotically unbiased (hence converge to the correct value as the number of data points grows arbitrarily large), though individual estimators in a consistent sequence may be biased (so long as the bias converges to zero); see bias versus consistency.
All else being equal, an unbiased estimator is preferable to a biased estimator, but in practice biased estimators are frequently used, generally with small bias. When a biased estimator is used, bounds of the bias are calculated. A biased estimator may be used for various reasons: because an unbiased estimator does not exist without further assumptions about a population or is difficult to compute (as in unbiased estimation of standard deviation); because an estimator is median-unbiased but not mean-unbiased (or the reverse); because a biased estimator gives a lower value of some loss function (particularly mean squared error) compared with unbiased estimators (notably in shrinkage estimators); or because in some cases being unbiased is too strong a condition, and the only unbiased estimators are not useful. Further, mean-unbiasedness is not preserved under non-linear transformations, though median-unbiasedness is (see § Effect of transformations); for example, the sample variance is an unbiased estimator for the population variance, but its square root, the sample standard deviation, is a biased estimator for the population standard deviation. These are all illustrated below.
0 Comments