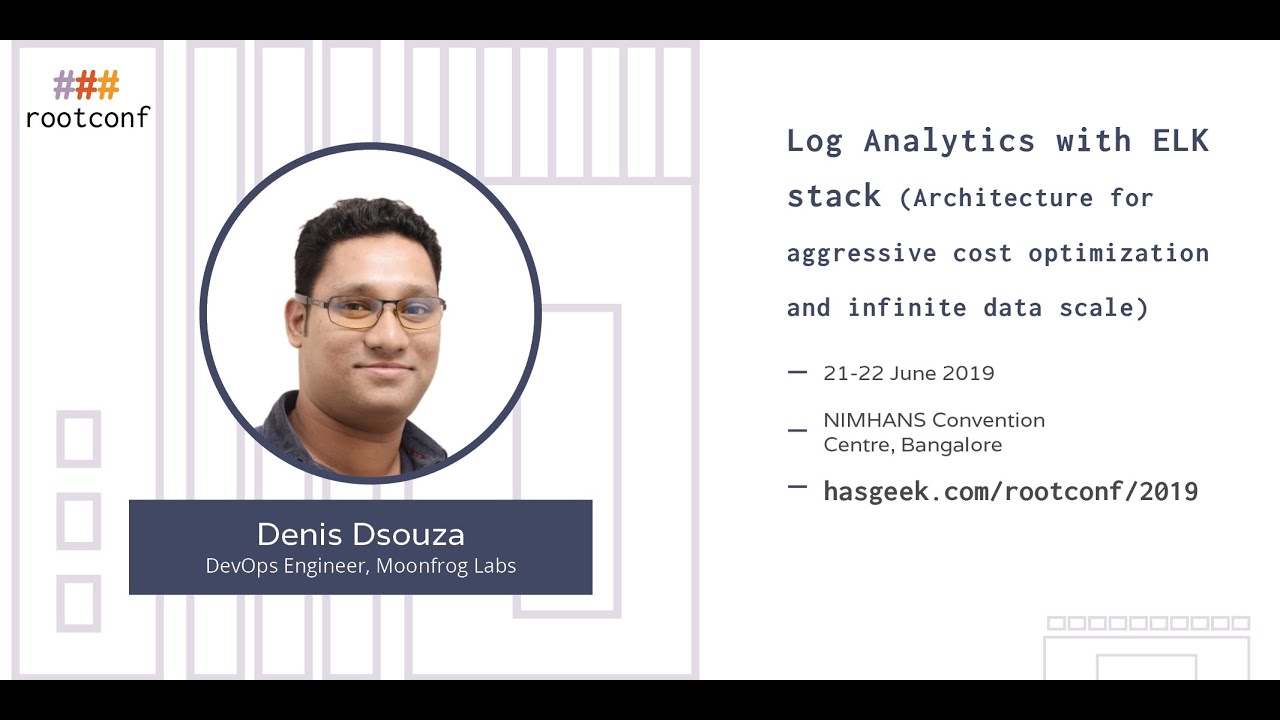
In this session, we will walk through various design choices we made to have a high performing log analytics cluster, aggressively optimized for cost and support for infinite data scale without exponentially increasing cost.
If you are planning on setting up or re-looking at your log analytics, this could be interesting to you.
Business Requirements/Use Cases
- Log analysis platform (Application, Web-Server, Database logs)
- Data Ingestion rate: ~300GB/day
- Frequently accessed data: last 8 days
- Infrequently accessed data: 82 days (90 - 8 days)
- Uptime: 99.90
- Hot Retention period: 90 days
- Cold Retention period: 90 days (with potential to increase)
- Cost effective solution
Areas of optimization
- Application
- Infrastructure
Cost Optimization
- Replica counts and its impacts
- How to run ELK on Spot instances correctly.
- EBS Costs can be high, how to set up Hot / Cold data storage
- Auto Scaling
- On-demand ELK Cluster
Infinite Data Retention
- How to setup S3 as a hot backup
- Recover on Demand
Numbers/Tradeoffs
- Cost/GB data ingested
- Trade-offs made
- DR mechanisms
Conclusion
- Building a log analytics is not rocket science. But it can be painfully iterative if you are not aware of the options. Be aware of the trade-offs you are OK making and you can roll out a solution specifically optimized for that.
Denis is a DevOps Engineer at Moonfrog Labs. He has over 6 years of experience and has worked with a variety of technologies in both service-based and product-based organizations.
0 Comments